Step-by-step, everything we need for nanosecond simulations.
In brief:
- The article discusses the main challenges we should face to run long-timescale (>>10 ps) nonadiabatic-dynamics simulations in full nuclear dimensionality.
Nonadiabatic dynamics simulations in the long timescale (much longer than 10 ps) are the next challenge in computational photochemistry. Several groups are already taking the first steps in this direction, mainly profiting from the computational acceleration due to machine learning algorithms.
Saikat Mukherjee, Max Pinheiro, Baptiste Deoulin, and I explore what do we need to take care of to run such dynamics and get reliable results. It’s not only a matter of accelerating the propagation. There are many other issues like how stable the integration is or can we use a method like surface hopping, developed for the ultrafast scale, to make predictions in the long timescale?
Forst, we delimit the scope of what we expect from methods to run such simulations. They should:
- work in full nuclear dimensionality;
- be general enough to tackle any type of molecule;
- not require unrealistic computational resources.
Bearing these constraints in mind, we examined the main methodological challenges we should face to advance the field:
- the computational costs of the electronic structure calculations;
- the stability of the integration methods;
- the accuracy of the nonadiabatic dynamics algorithms;
- software optimization.
We discussed each of these issues based on simulations of model systems and actual molecules.
Computational costs
We analyzed three strategies to speed up electronic structure calculations, using parameterized and approximated methods, employing model Hamiltonians, and predicting electronic properties with machine learning.
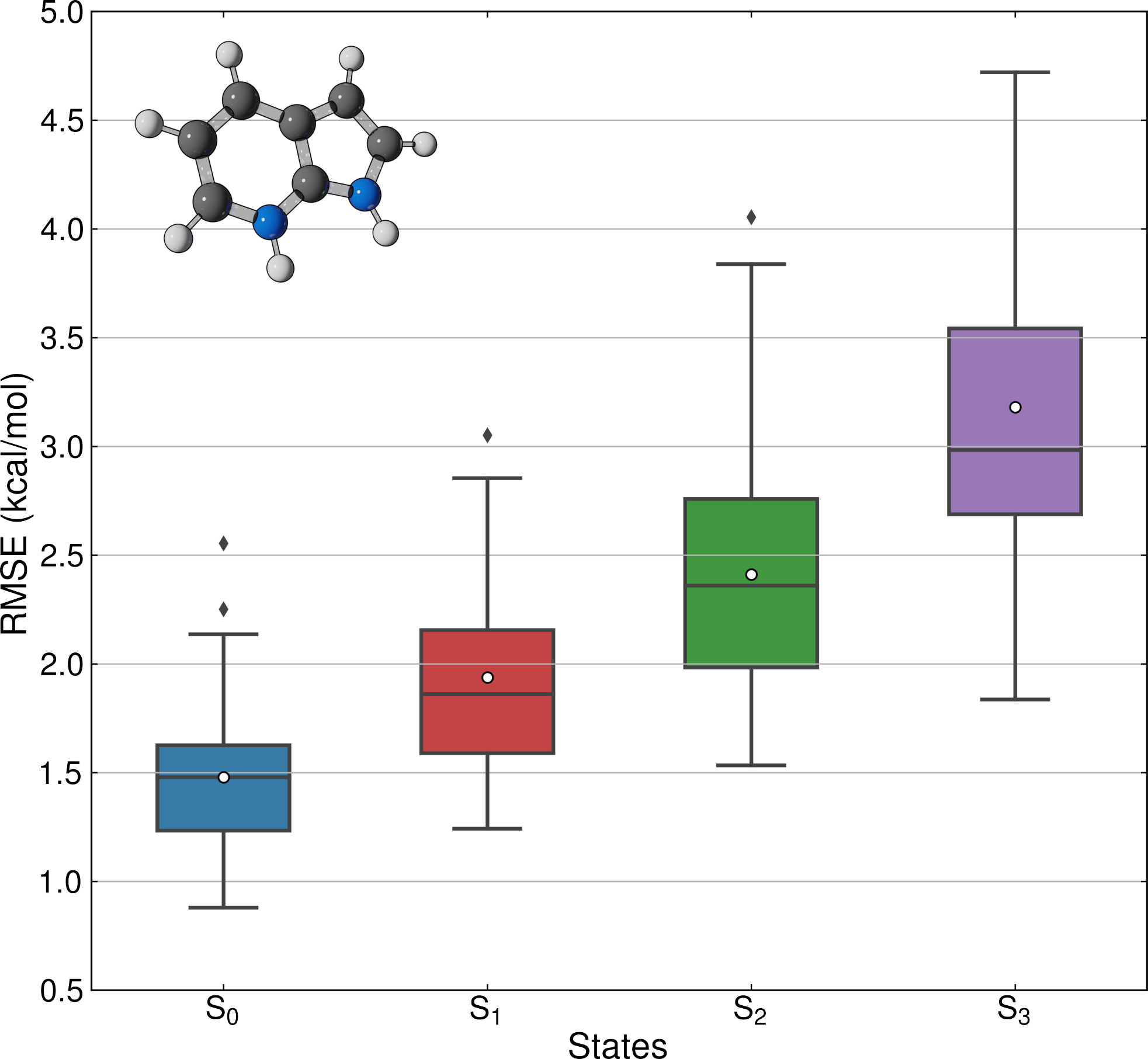
Assessment of KREG model uncertainty for predicting ground- and excited state energies of an entire surface hopping trajectory of protonated 7-azaindole.
The first strategy is consistently associated with strong accuracy loss. The second has the main challenge of parameterizing full-dimensional systems, a procedure that has found an ally in machine learning. For the third strategy, we believe machine learning may be on the verge of becoming a crucial element for long-timescale dynamics. Nevertheless, it still misses clear protocols for training excited-state energies of large conformational sets and high density of states with the accuracy needed for dynamics.
Stability of integration methods
We showed that conventional methods for integrating the classical equations of motion should be adequate to extend simulations up to 1 ns, as long as the precision of the electronic structure properties is kept under control.
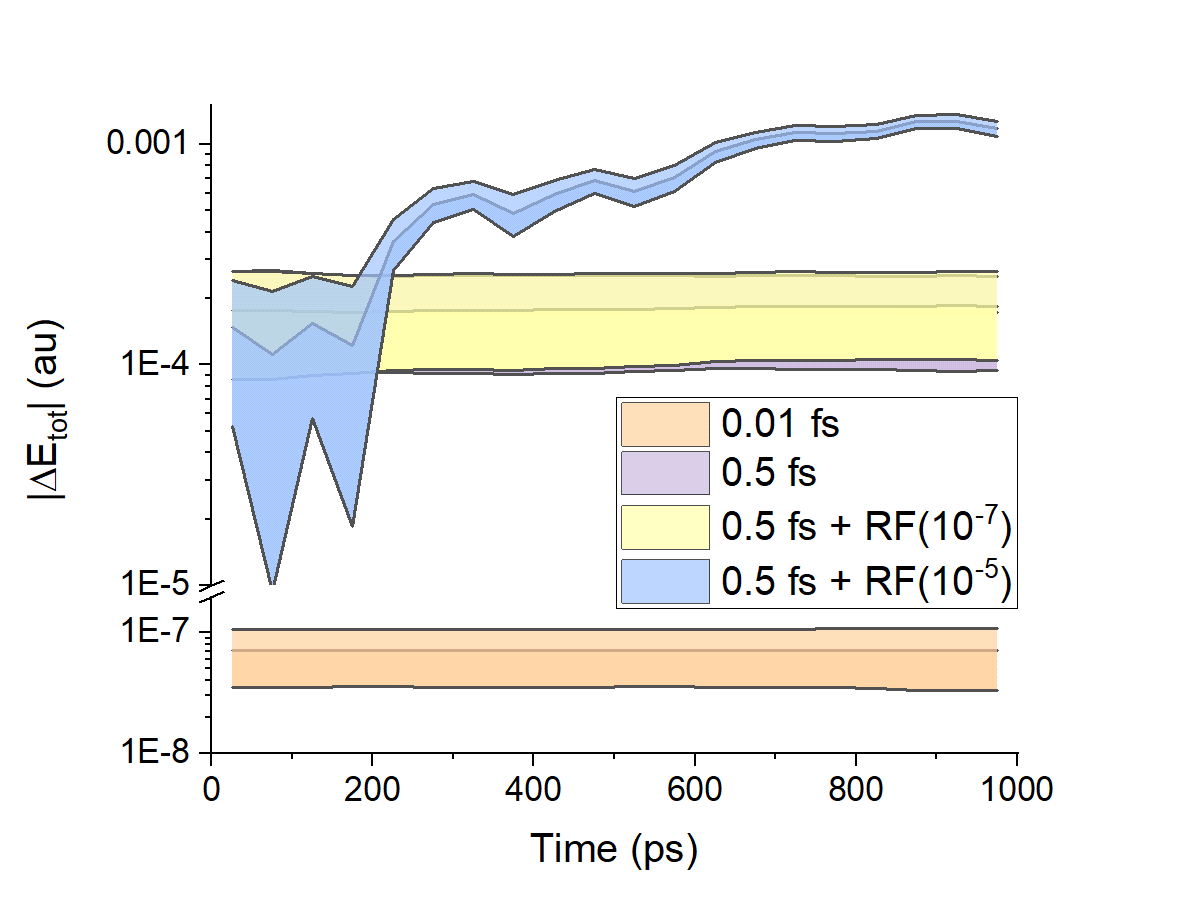
Distribution of total energy deviation as a function of time for different integration setups. RF are random fluctuations added to the forces.
Moreover, ZPE leaking must be accounted for. Hessian-free methods for correcting ZPE leaking must be developed. (And we are working on that.)
Accuracy of the nonadiabatic dynamics algorithms
We evaluated the performance of decoherence-corrected fewest-switches surface hopping (DC-FSSH) in the long timescale compared to MCTDH. The results for internal conversion in the weak-coupling regime show that it can make predictions in semi-quantitative agreement with wavepacket propagation. However, we still need to go deeper into these tests.
Software optimization
Finally, we also described our optimization of the Newton-X program to reduce computational overheads in processing and data storage.
We completely redesigned Newton-X. This new version, termed Newton-X NS (novel series), is characterized by improved performance, big-data management, and compliance with open data standards.
In the most favorable case, computational time in Newton-X NS can be reduced more than 1000 times compared to the costs of simulations done on the classical Newton-X (now renamed Newton-X CS for classical series).
This article is part of the Philos. Trans. theme issue ‘Chemistry without the Born–Oppenheimer approximation’ edited by Federica Agostini and Basile Curchod.
MB
Reference
[1] S. Mukherjee, M. Pinheiro Jr, B. Demoulin, M. Barbatti, Simulations of molecular photodynamics in long timescales, Philos. Trans. R. Soc. A 380, 20200382. (2022), DOI: 10.1098/rsta.2020.0382